Generalized Linear Models & Why Statisticians Should Not Be Allowed to Name Things
Statisticians are good at lots of things but naming is not one of them. If Carl Linnaeus had been a statistician the name for camel would be Horse With Hump and for elephant Really Big Horse with Nose based on the fact that both have four legs and people ride them.
Such is the case with the Generalized Linear Model.
Let’s start with the General Linear Model, because it is easier. As I said before, the General Linear Model is general (and also linear and a model). Then I said this “Almost all questions that can be stated:
Is there a relationship between this thing and this other thing?
….and rambled on a bunch.
So, now that we have that down pat … there is the GENERALIZED Linear Model because the general linear model was not general enough for us. You see GLM (well, both are GLM so let’s call it the horse), was based on the assumption that the errors follow a multivariate normal distribution. So a Generalized Linear Model (let’s call it the camel), generalizes from our basic GLM (the horse) to other types of distributions.
Then there are General Linear MIXED models (maybe those are zebras ?).
The basic Analysis of Variance, going back to the original apparently not-so-general-as-originally-believed model, is quite simple. You have one (or more) independent variables that can be broken down into two (or more) groups. Let’s say gender. You have people in two groups, male and female. This is not a sample of all genders. It’s all the genders there are. The same is true if you put people in an experimental and a control group. This is NOT a mixed model, because you only have one type of effect. Hurray you.
You can have a random effects only model. This example from Stanford looks at whether there is variability across brands of beer. With brands as the random effect, eight different measures are taken across six brands of beer. This is a very worthwhile study as it involves drinking 48 bottles of beer. Oh, and repeated measures, too.
What if you had taken 20 people and put ten in one group that got to drink beer and ten in another group and tested them four times, with the first group getting to drink two beers between each testing and the second group having to watch Sarah Palin videos? Now you have a random effect. You do not have all possible levels of people. You have randomly sampled twenty out of the population. There will be an effect of person and an effect of group. So, this is a random effect AND a fixed effect in your model. I presume your dependent variable will be stupidity with the research question what makes you become stupider, listening to Palin or getting drunk. I’m agnostic on this question.
If you have a MIX of fixed and random effects, then it is a mixed model.
Generalized Linear Mixed Models are perfectly cool with heterocatanomic multivariate distributions, that is when you have some predictor variables that have one distribution, say a Poisson distribution, and another that has a normal distribution.
Then, just when you thought it was safe to go read something else, there are Nonlinear mixed models.
My husband asked if there was such a thing as model mixers, where statisticians got to go to parties and mix with models like Tyra Banks.
Lovely daughter number two mutters,
“Have you seen what the people Mom hangs out with LOOK LIKE? The best you could say for any of them is they are good-looking for old people who work on computers all day. So, for model mixers, I’m going with – No.”
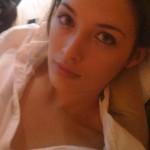
this the funniest and clearest description of various stat models that I have ever read. And your husband and daughter are hilarious.
Your daughter would get along with mine.
I love this blog.
Aaaw, thank you!